Diagnosis of Intraductal Papillary Mucinous Neoplasms (IPMN) using Deep Learning (ISBI 2018)
Abstract:
Pancreatic cancer has the poorest prognosis among all cancer types. Intraductal Papillary Mucinous Neoplasms (IPMNs) are radiographically identifiable precursors to pancreatic cancer; hence, early detection and precise risk assessment of IPMN are vital.
In this work, we propose a Convolutional Neural Network (CNN) based computer aided diagnosis (CAD) system to perform IPMN diagnosis and risk assessment by utilizing multi-modal MRI. In our proposed approach, we use minimum and maximum intensity projections to ease the annotation variations among different slices and type of MRIs. Then, we present a CNN to obtain deep feature representation corresponding to each MRI modality (T1-weighted and T2-weighted). At the final step, we employ canonical correlation analysis (CCA) to perform a fusion operation at the feature level, leading to discriminative canonical correlation features. Extracted features are used for classification.
Our results indicate significant improvements over other potential approaches to solve this important problem. The proposed approach doesn’t require explicit sample balancing in cases of imbalance between positive and negative examples. To the best of our knowledge, our study is the first to automatically diagnose IPMN using multi-modal MRI.
Method:
In this work, we hypothesize and evaluate the influence of complementary information in T1-weighted and T2-weighted scans that can be utilized to perform improved diagnosis of IPMN.
We employ multiple imaging modalities of MRI (T1 and T2) and fuse the feature representation using Canonical Correlation Analysis (CCA) to obtain better discrimination between normal and subjects with IPMN. We also perform further stratification of IPMN in low-grade and high-grade categories.
First, the minimum and maximum intensity projections are computed corresponding to T1 and T2 scans respectively. The intensity projections are then fed into a pre-trained Convolutional Neural Network (CNN) to obtain feature representation. Canonical Correlation Analysis (CCA) based feature fusion is performed in order to obtain discriminative and transformed feature representation. Finally, an SVM based classifier is employed to obtain the final label (normal or IPMN).
We perform extensive experimental evaluations are performed on a dataset comprising 139 subjects, the largest study of IPMN to date.
Results:

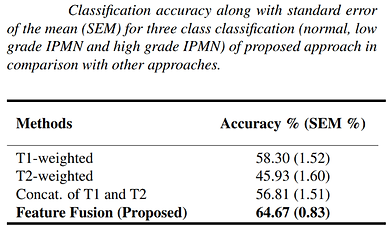
An overview of the proposed approach

Qualitative results of our proposed approach, showing minimum and maximum intensity projected images for T1 and T2 scans on left and right respectively. Each row represents a different case where the images correctly classified as IPMN are shown in green, whereas the misclassification of IPMN as normal are shown in red.
Related Publication/Reference:
"Deep Multi-Modal Classification of Intraductal Papillary Mucinous Neoplasms (IPMN) with Canonical Correlation Analysis", Sarfaraz Hussein, Pujan Kandel, Juan E. Corral, Candice W. Bolan, Michael B. Wallace, Ulas Bagci. IEEE International Symposium on Biomedical Imaging (ISBI) 2018 (accepted). [Paper]