Computer aided Detection of White and Brown Fat from Radiology Scans
Introduction:
Obesity is one of the most prevalent health conditions in the world. In this work, we investigate the automatic detection of white and brown adipose tissues using Positron Emission Tomography/Computed Tomography (PET/CT) scans, and develop methods for the quantification of these tissues at the whole-body and body-region levels.
In the first module, we detect white adipose tissue (WAT) and its two sub-types from CT scans: Visceral Adipose Tissue (VAT) and Subcutaneous Adipose Tissue (SAT). In the second module, we automatically detect, segment, and quantify brown adipose tissue (BAT) using PET scans because unlike WAT, BAT is metabolically active.
Both modules are integrated via an automatic body-region detection unit based on one-shot learning. Experimental evaluations conducted on 151 PET/CT scans achieve state-of-the-art performances in both central obesity as well as brown adiposity quantification.
Method:
First, we propose an automated abdominal and thorax region detection algorithm, based on deep learning features. Second, we develop an unsupervised learning method for separating VAT from SAT using appearance (via Local Outlier Scores) and geometric (via Median Absolute Deviation) cues. For volumetric quantification, we integrate contextual information via a sparse 3D Conditional Random Fields (CRF) based label fusion algorithm. Our work can be considered as the largest central obesity quantification study (151 CT scans) to date, validating accurate region and abdominal fat detection algorithms.
For BAT detection and segmentation, we first use a fixed HU interval to identify total adipose tissue (TAT) from CT images. Next, we devise a seed sampling scheme for extracting foreground and background cues from high uptake regions of PET images in head-neck and thorax regions only. The identified seeds are propagated into the corresponding CT scans as well. This is followed by a PET guided image co-segmentation on the hyper-graph (PET/CT) to delineate potential BAT regions. Lastly, for FP (False Positive) rejection, we propose a novel probabilistic metric that combines Total Variation and Cramer-von Mises´ distances to differentiate BAT regions from non-BAT regions. Figure 2 shows the overview of the proposed system.
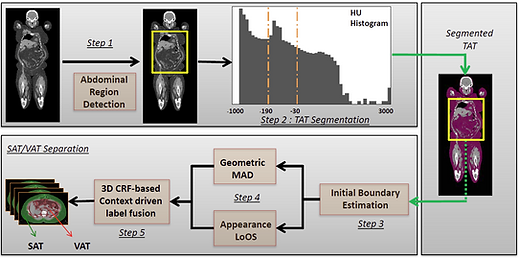
An overview of the proposed SAT-VAT separation method.
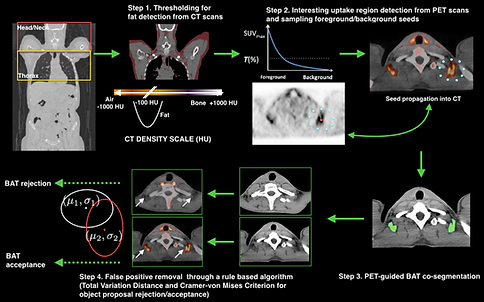
An overview of the proposed Brown Fat segmentation method.
Results:

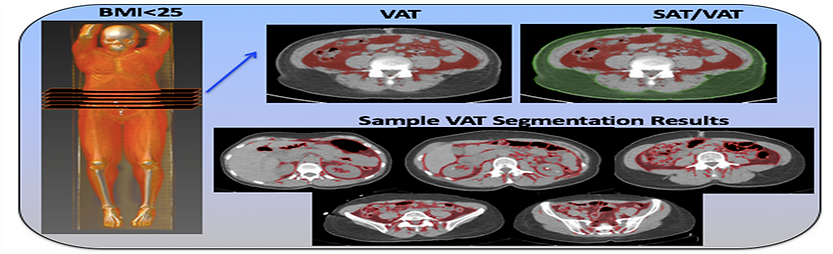
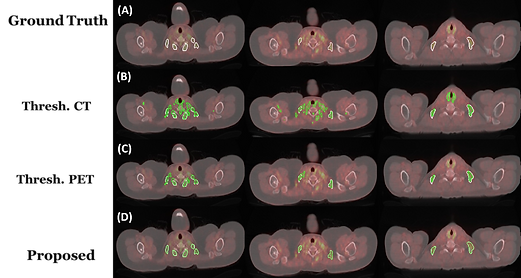

SAT-VAT qualitative results for subjects with BMI < 25 (left) and BMI > 30 (right)
SAT-VAT quantitative results using Dice similarity scores and Mean Absolute Error (in mL)
Qualitative results for Brown Fat Segmentation in comparison with threholding on CT and thresholding on PET scans
Poster: [Download]
Related Publication/Reference:
"Automatic Segmentation and Quantification of White and Brown Adipose Tissues from PET/CT Scans", Sarfaraz Hussein, A.Green, A.Watane, D.Reiter, X.Chen, G.Z.Papadakis, B.Wood, A.Cypess, M.Osman, Ulas Bagci. IEEE Transactions on Medical Imaging [Paper]
BibTeX:
@article{hussein2017automatic,
title={Automatic segmentation and quantification of white and brown adipose tissues from PET/CT scans},
author={Hussein, Sarfaraz and Green, Aileen and Watane, Arjun and Reiter, David and Chen, Xinjian and Papadakis, Georgios Z and Wood, Bradford and Cypess, Aaron and Osman, Medhat and Bagci, Ulas},
journal={IEEE Transactions on Medical Imaging},
volume={36},
number={3},
pages={734--744},
year={2017},
publisher={IEEE} }